Making the most of the CI opportunity
Collective intelligence (CI) is powered by mobilising our collective knowledge and resources to solve problems. Yet, anyone who has tried to organise the effective exchange of ideas in something as simple as a meeting knows how difficult it can be to do it well. Creating larger-scale and looser online communities that share resources, tasks and goals is even more complicated. After all, there were at least seven failed attempts to create online collaborative encyclopedias before the success story of Wikipedia.
Making deliberate choices that optimise the collective power of groups is known as collective intelligence design. Below we outline some of the key principles that underpin CI and the challenges CI projects often face.
Crowds need to be diverse and get value from participation
One of the most important principles to help groups become more than the sum of their parts is diversity. For example, Anita Woolley has demonstrated that gender diversity helps smaller groups to improve their problem-solving ability and that cognitive diversity is vital for creativity and learning in workplace teams. The principle holds even when it comes to larger-scale CI efforts, where it may intuitively seem that diverse contributions could make it harder to distinguish signal from the noise. This is because the ‘wisdom of crowds’ relies on everyone making mistakes in slightly different ways. For example, in crowd predictions, if some people have a tendency to overestimate, while others are more likely to underestimate, overall their errors cancel each other out, making collective estimates more robust to interference from individual biases.
Another core principle of CI is making sure that the overall goal of the project brings some benefit to the participants, so that they are motivated to contribute. In citizen science, projects tend to offer a range of incentives, such as learning new skills or socialising with peers, which help to attract high-quality contributions from the community members. Allowing people to contribute their views equally and independently, and selecting the most appropriate methods for aggregating diverse inputs for the problem at hand are also important features of successful CI projects. For example, deliberative democracy methods that involve citizens in decision‑making may choose to adopt the final preferences of the majority or require the group to reach a consensus.
CI design challenges
Even though research has helped us to understand which principles are important for CI, operationalising them can still be challenging. The most common challenges that stand in the way of making the most of CI approaches to solving problems fall into three broad categories:
1) Making sense of the data - The big data at the heart of many CI projects is a double-edged sword. It can help drive better-informed actions and decisions, but without efficient systems for aggregating, organising and combining this data, there is also a risk of getting lost in the noise. Data for CI projects often comes from novel sources, like citizen-generated content on social media, mobile phone call records or satellite images, which require novel approaches to analysis in order to extract insights. Sometimes the data is of variable quality or, in the case of digital democracy projects, may require the integration of multiple competing viewpoints. Organising and prioritising this data to make it searchable and usable towards the goal of a project can be a difficult task for public sector and civil society organisations looking to use CI to address social challenges.
2) Setting the right rules for exchanging information and skills - Realising the benefits of a group’s diversity is only possible when there are effective mechanisms for people to access information and skills. The rules governing how group members interact with one another impacts how easily ideas spread and take hold in the group. For example, well-timed breaks to achieve a balance between group work and exploring ideas at the individual level encourage productivity in problem-solving. The structure of a community network can play an important role in determining whether a group is able to exchange information efficiently. CI also relies on having shared open repositories of knowledge, accessible to old and new members alike. Well-known online platforms like GitHub or Wikipedia are often used by communities to document their processes. These function as a source of collective memory, and their absence can lead to the repetition of old mistakes over time.
3) Overcoming human cognitive biases - All people can be subject to biases, which affect their interactions with other people. In certain circumstances these biases are useful; they provide shortcuts[1] to help us manage our cognitive resources more efficiently. However, some social biases can interfere with the principle of diversity. Status and power imbalances between participants can silence those who are lower in the social hierarchy and confirmation bias can lead to certain ideas being devalued because they do not match assumptions held by the group. Apart from biases, some fundamental properties of human cognitive architecture can prevent us from contributing effectively to CI projects. For example, due to limits in working memory and attention, we struggle to keep more than a small number of ideas in mind at any one time and can get easily distracted. These limits are put under even more pressure in CI projects where there is a lot of information to process.
In practice, many of these challenges overlap and can even exacerbate each other. For example, the limits of human attention may be stretched by poor platform infrastructure that overwhelms participants with too much data and information, making it difficult to make meaningful connections and build on the work of others. In recent years, experiments in CI design have expanded to include AI in order to address one or more of the challenges outlined above.
How different AI tools can enhance CI
The field of AI comprises a range of methods, at varying levels of maturity, for implementation in real-world contexts. Focusing specifically on the methods that are already being used to enhance CI we have identified broad application areas, which we refer to as ‘types of AI’. Below, we define each of the types alongside the data they rely on as input and examples of how they are being used to solve problems in practice.
AI is being implemented across a range of CI methods, from citizen science to crowdsourcing and digital democracy platforms. Many well-established CI initiatives, such as the Humanitarian OpenStreetMap Team and Zooniverse, which bring together distributed volunteers for humanitarian action and scientific research respectively, have only started implementing AI into their community platforms in the past couple of years, after existing for a long time without it. Others, like Factmata and Wefarm, which crowdsource expertise to identify misinformation and help farmers to solve problems respectively, have been created as integrated AI & CI platforms from the outset. We also found examples that started with the technology first, such as Swarm AI, where the AI works in real time with human groups to answer questions and make predictions. Swarm AI can be used by any group or team, but it does not have a dedicated community of practice attached to the tool.
It is difficult to carry out a comprehensive mapping of either CI or AI projects separately, let alone together. Most existing examples do not document their use of methods in detail or give insight into any other approaches they considered. Thus, while we have tried to capture the breadth of the field, we cannot claim to be exhaustive. Further progress will require much more transparency and sharing of lessons between all of the actors in the AI & CI ecosystem.
Types of AI
The ability of a computer to understand, analyse or generate images and/or videos.
Type of data accepted as input:
- User-generated** images and videos
- Satellite images
- Drone images
Case studies:
SyrianArchive, MapwithAi, iNaturalist, OneSoil, WeRobotics, Decode Darfur
** User-generated data inputs can be either actively submitted by participants of a CI initiative or collected by scraping online content and other public information.
The ability of a computer to understand and translate human-generated text and potentially simulate language.
Type of data accepted as input:
- User-generated** text on social media and blogs
- Transcribed documents
Case studies:
WeFarm, Factmata, Dataminr, Siminchikkunarayku
** User-generated data inputs can be either actively submitted by participants of a CI initiative or collected by scraping online content and other public information.
The ability of a computer to recognise, analyse, manipulate and potentially generate speech or audio signals.
Type of data accepted as input:
- User-generated** audio
- Sensor data
Case studies:
Common Voice, Siminchikkunarayku, Pulse Lab Kampala
** User-generated data inputs can be either actively submitted by participants of a CI initiative or collected by scraping online content and other public information.
The ability of a computer to analyse structured data using numeric and machine-readable data.
Type of data accepted as input:
- Official data (e.g. databases)
- Structured taxonomies
- Participant rankings
Case studies:
Early Warning Project, Zooniverse (Gravity Spy), Polis, Epistemonikos
*Predictions on structured data and recommender systems both rely on algorithms from classical machine-learning. See Figure 1 for an overview of AI methods being used to enhance CI.
A system where many individual (software) agents react to their local environment and other agents to produce emergent collective behaviour.
Type of data accepted as input:
- Data on past performance
Case studies:
Swarm AI, Malmo Collaborative AI
A system situated within and as part of an environment that senses and acts on that environment.
Type of data accepted as input:
- Human teleoperation
- Video demonstrations
Case study:
RoboTurk
A subclass of information filtering system that seeks to predict the ‘rating’ or ‘preference’ a user would give to an item.
Type of data accepted as input:
- Participant rankings
- User-generated** images, videos and text
Case study:
CAT Lab
*Predictions on structured data and recommender systems both rely on algorithms from classical machine-learning. See Figure 1 for an overview of AI methods being used to enhance CI.
** User-generated data inputs can be either actively submitted by participants of a CI initiative or collected by scraping online content and other public information.
Case studies:
Dataminr, Decision Theatre
Explore 20 projects that bring Artificial Intelligence and Collective Intelligence together
Artificial intelligence and collective intelligence: case studies
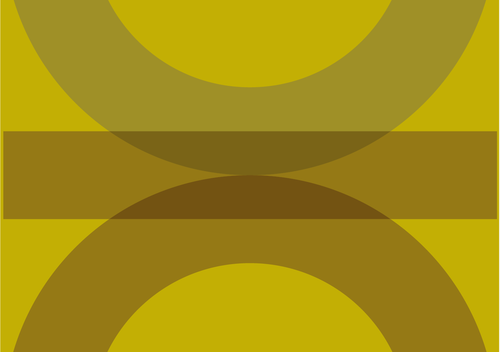
Added value from AI
Where AI is already enhancing CI, the value it adds to the collective can be broken down into eight broad categories. These categories address challenges commonly encountered in CI, such as those related to managing data, process and people (see CI design challenges above). These different sources of added value demonstrate that AI functions as a cognitive tool that allows the extension of natural intelligence, from perception and decision-making to creativity and learning. Much like the transition to literacy (both handwritten and print) from predominantly oral cultures first amplified individual and collective intelligence for people, by externalising memory and extending the reach of ideas,[1] the added value that smart algorithms bring to CI comes in many different forms.
Download the added value of AI
- Growth in efficiency and scale of data processing: Combining and processing vast amounts of structured and unstructured data to enable quicker response.
- Making new causal connections for complex issues: Identifying new patterns and relationships between variables in high-dimensional datasets, either to infer causality or make predictions.
- Organising human knowledge and finding structure: Filtering, clustering and ranking information and helping to group data into categories.
- Optimising and prioritising processes: Streamlining complex systems and processes to optimally satisfy different (competing) resource constraints.
- Modelling and visualising complexity: Creating models of entire systems to expand our capacity for systems thinking, multi-stage processing and an individuals’ role within a collective.
- Increasing the reach of and access to rare knowledge: Using rare knowledge and non-traditional data sources to codify specialised expertise and gain new insights.
- Transformational creativity:[2] Expanding the boundaries of the solutions normally considered by humans, generating new ideas or helping to surface old ideas.
- Improving interactions between individuals: Optimising individual contributions and facilitating knowledge exchange between members of a group in real time.
How AI can help address CI project challenges
The table below summarises some of the most common design challenges faced by CI projects, as outlined in Section (see CI design challenges above). Drawing on the tools currently being used, we provide examples of the potential opportunities offered by AI methods to overcome these challenges and enhance CI.
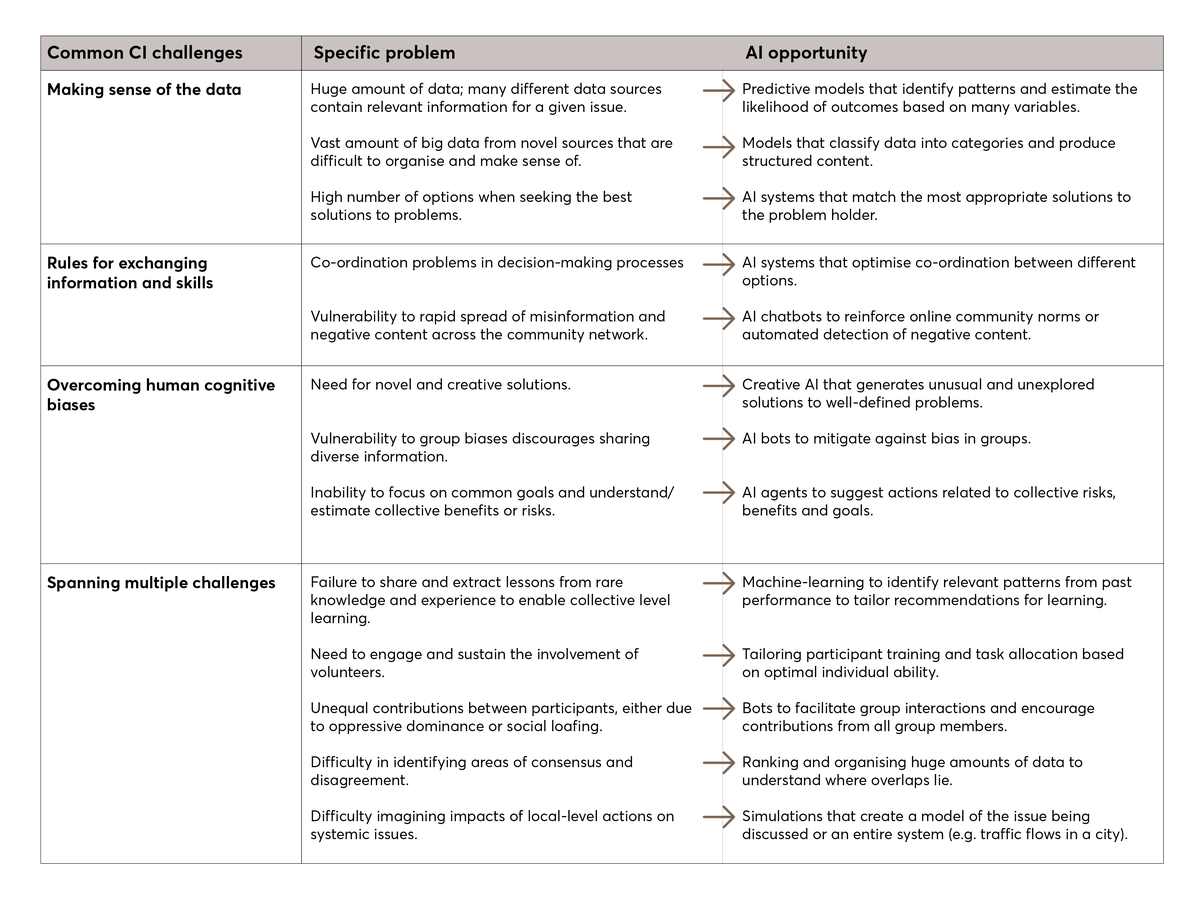
[1] Known as heuristics.
[2] This term was first introduced by Margaret Bowden.