Here, we focus on the knowledge base for artificial intelligence (AI) research to tackle COVID-19. We are particularly interested in determining the extent to which AI draws on subject expertise from medicine and the biological sciences as well as computer science disciplines. In order to explore this question, we have extracted information about 22,404 papers cited by around 3,000 papers in our COVID-19 corpus.
We begin by comparing the share of citations to papers in various high-level disciplines from AI and non-AI research. Figure 8 presents the share of all citations from AI and non-AI COVID-19 papers accounted for by papers in various fields of study at the highest level of aggregation in Microsoft Academic Graph’s taxonomy.
It shows that computer science papers account for almost half of the citations from AI papers (five times higher than the share outside of AI). By contrast, the share of citations to medicine from AI papers is 35 per cent lower than in non-AI papers and the share of citations to biology papers is 65 per cent lower. This suggests that AI COVID-19 research draws on a different body of knowledge from non-AI research. Further confirming this idea, we find that AI and non-AI research to tackle COVID-19 only share 3.7 per cent of their references.
Figure 8: AI research tends to draw strongly on computer science, and much less on other disciplines such as medicine and biological sciences
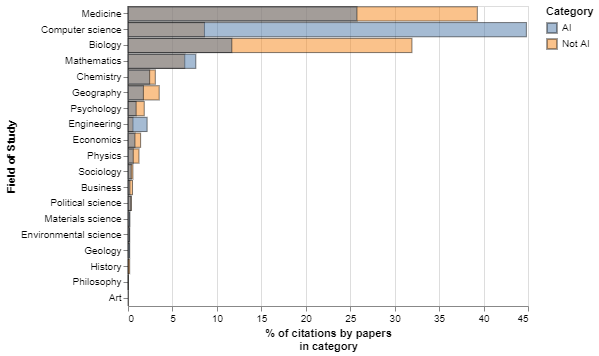
An interactive version of this graph is available in the online report
The result we reported above could reflect specialisation of AI and non-AI research in different topics drawing on distinct bodies of knowledge. To account for this, we compare the citation propensities of AI and not AI papers in the same topic, drilling down one level further from disciplines into subfields of research. We show the results in Figure 9.
There, we compare the share of citations to different sub-fields of study by AI and non-AI papers in different topical clusters. We focus on topical clusters with high levels of AI activity and on the 30 most highly cited fields of study in the COVID-19 corpus of research We colour the points in the chart to represent the higher-level field of study to which each subfield belongs.
Figure 9: AI and non-AI papers cite different subfields of research even when they are in the same topic
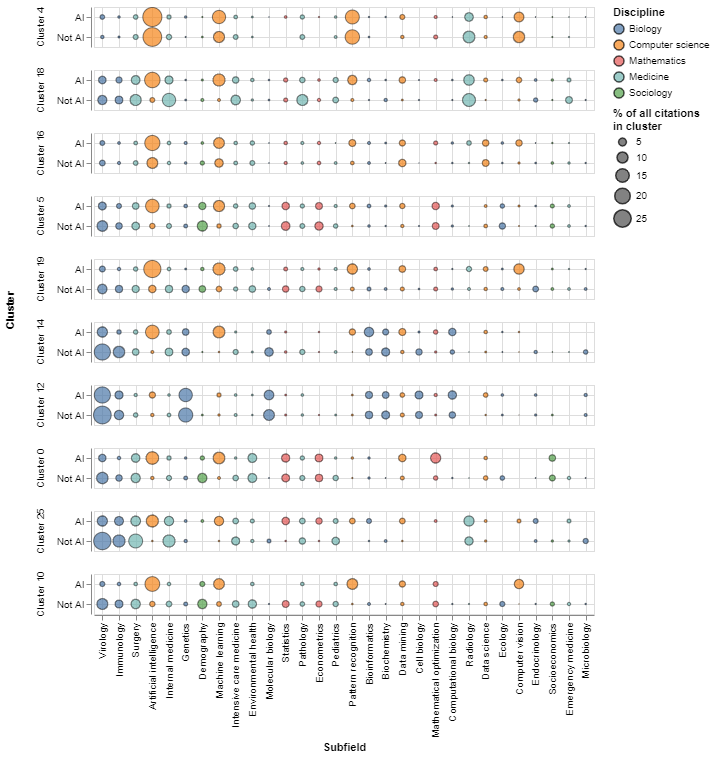
An interactive version of this graph is available in the online report
The figure shows important differences in cited subfields between AI and non-AI research, even inside the same topical cluster. For example, non-AI papers in cluster 19 focus their citations much more strongly on internal medicine, intensive care medicine and pathology while AI focuses on machine learning, pattern recognition and computer vision. In cluster 14 (about drug discovery), we see that a larger share of non-AI citations go to immunology, surgery and internal medicine, and molecular biology.
Consistent with this, and as Figure 10 shows, we find that the levels of overlap between papers cited in AI and non-AI research in the same topical cluster tend to be low, ranging between 5-8 per cent for computer vision papers and zero for several clusters (admittedly, some of these do not include any AI papers).
Figure 10: There are very low levels of overlap between the AI and non-AI references in different topical clusters
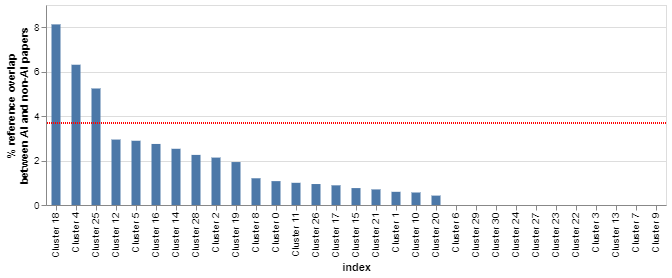
An interactive version of this graph is available in the online report